Synergizing Human Insight and Machine Learning for Enhanced Pharmacovigilance Literature Monitoring
- 10/08/2023
Patient safety and drug efficacy are paramount concerns in the dynamic realm of pharmacovigilance. A novel era of vigilance arises from fusing machine learning and human expertise. An essential facet of this partnership is the surveillance of medical literature for adverse events, drug interactions, and emerging safety issues. This article delves into the dynamic interplay between machine learning algorithms and human professionals, explicitly focusing on their synergy in enhancing the safety of pharmaceutical products.
Harnessing Machine Learning for Literature Monitoring
Machine learning, an arm of artificial intelligence, has emerged as a powerful tool in pharmacovigilance literature monitoring. These algorithms can rapidly analyze vast amounts of medical literature from diverse sources. By leveraging these capabilities, machine learning offers several advantages:
1. Swift Data Processing. Machine learning algorithms can process an unprecedented volume of medical literature in a fraction of the time it would take humans. This speed ensures the timely identification of emerging safety concerns.
2. Pattern Recognition. By discerning patterns humans might overlook, machine learning algorithms can identify subtle correlations between drugs and adverse events, leading to more comprehensive safety assessments.
3. Real-time Insights. With the ability to operate in real-time, machine learning can swiftly detect signals of adverse events, enabling prompt interventions and regulatory actions.
4. Data-driven Prioritization. Machine learning can prioritize literature findings based on factors such as severity and frequency of adverse events, assisting human professionals in focusing their efforts where they matter most.
An example from pharmacovigilance practice
Imagine yourself with the latest medical journal – a repository of knowledge. However, scrolling through 100-300 pages for information about drug safety takes 5 to 15 minutes. And this is without even reading the text. While you’re just leafing through a magazine, the DrugCard platform scans several journals for drug-related keywords and prepares search results. It’s fantastic, but the DrugCard platform only needs 0.5-1.5 seconds to process one page of text. In other words, the DrugCard platform can analyze a 200-page journal in just 1.5-5 minutes.
Human Expertise: A Vital Component
While machine learning is a powerful ally, the human touch remains indispensable in pharmacovigilance literature monitoring. Therefore, the speed of machine learning analysis can only be imagined with pharmacovigilance specialists. Human expertise contributes a depth of understanding that machines cannot replicate:
1. Contextual Interpretation. Human experts bring a nuanced understanding of medical context, enabling them to distinguish between mere coincidences and genuine adverse reactions.
2. Clinical Judgment. Professionals with clinical experience can identify subtle nuances in adverse event descriptions, ensuring that the severity and relevance of events are accurately assessed.
3. Regulatory Compliance. Human experts are well-versed in regulatory frameworks, ensuring that adverse events are appropriately classified, reported, and managed following standards.
4. Complex Case Analysis. In cases where adverse events are convoluted or multifactorial, human professionals excel in deciphering the underlying causes and implications.
The Synergy: Machine Learning and Human Collaboration
The true power of pharmacovigilance literature monitoring is unlocked when machine learning and human expertise collaborate harmoniously:
1. Data Quality Enhancement. Human experts curate and validate the data used to train machine learning algorithms, ensuring the algorithms learn from reliable, accurate information.
2. Algorithmic Oversight: Human professionals supervise the machine learning algorithms, ensuring their output aligns with clinical reality and regulatory requirements.
3. Continuous Learning and Improvement. As human experts analyze the outcomes of machine learning algorithms, they can refine their approach and provide feedback for ongoing algorithm improvement.
Conclusion
In the pharmacovigilance world, combining machine learning’s computational prowess and human expertise’s discernment leads to more comprehensive, accurate, and efficient literature monitoring. A prime example of this synergy is the DrugCard platform, accelerating the process even further. As technology advances, this collaboration will undoubtedly play a pivotal role in ensuring pharmaceutical products’ safety and effectiveness, safeguarding patients’ health and well-being worldwide.
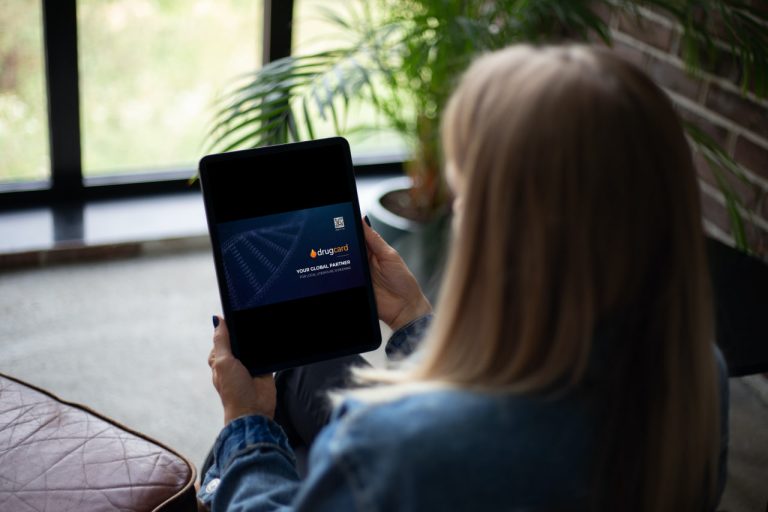
- 24/03/2025
- Drug Safety